This website uses cookies so that we can provide you with the best user experience possible. Cookie information is stored in your browser and performs functions such as recognising you when you return to our website and helping our team to understand which sections of the website you find most interesting and useful.
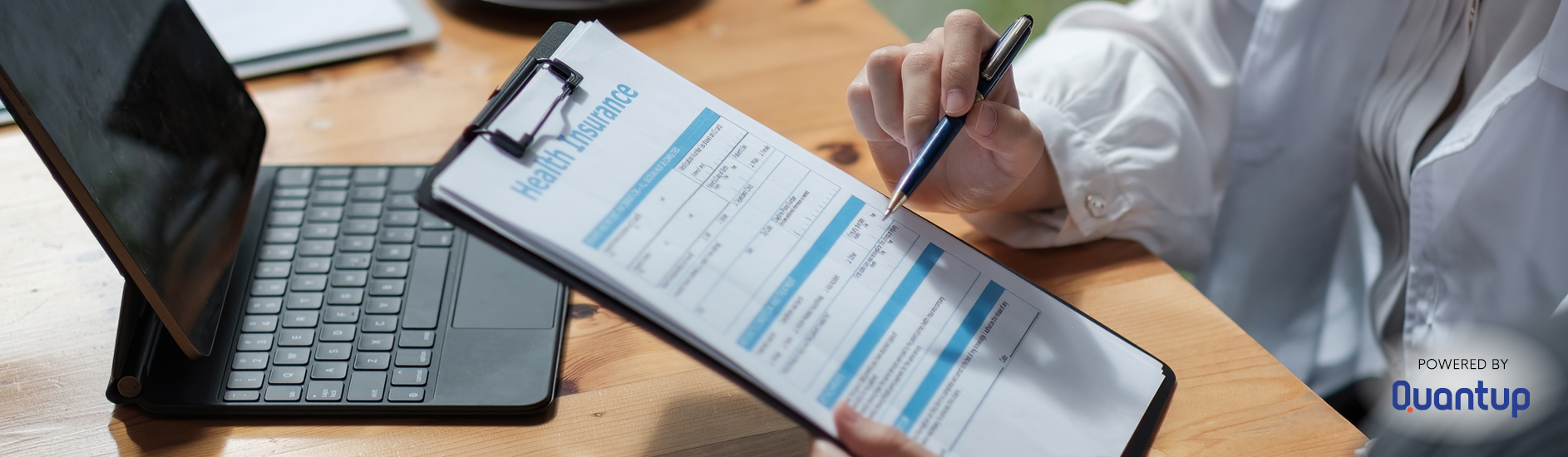
Customer
For one of the largest insurers in Poland, which is creating innovative solutions for the market, we performed a project in which we applied machine learning algorithms. It ended with considerable success and prestigious industry awards for the client.
Challenge
Compensation claims generally occur when there is an actual accident. Unfortunately, there are also attempts at fraud. In such a situation, you need to decide whether to refuse payment within a short period of time. An analysis must be carried out before refusing to pay compensation due to a suspected fraudulent claim.
Until now, the decisions were made by experts, with partial support from algorithms. They performed the first step using expert rules or a model, but the last step was always manual verification. However, poor algorithms meant that experts had to review many cases where the percentage of actual scams was small. The difficulty in finally identifying bad and good customers made the process inefficient.
The client’s team needed specialists to build good algorithms—one that would pass on for further review only cases with a high probability of being scams. By focusing only on these carefully selected cases, experts could get a better feel for them and decide whether to withhold or pay compensation.
Solution
The project involved nine people, including three specialists from the client’s team. We used machine learning (ML) algorithms based on historical data and in-depth process analysis to create a strong model.
The process of creating the AI model consisted of the following steps:
- Process analysis in the form of product workshops
- Provide data and analyze its quality/correctness
- Construction, delivery, and integration of the first models
- Feedback on the results provided
- Iterations that allow incremental improvements to the solution: data, models, and integrations with the existing system
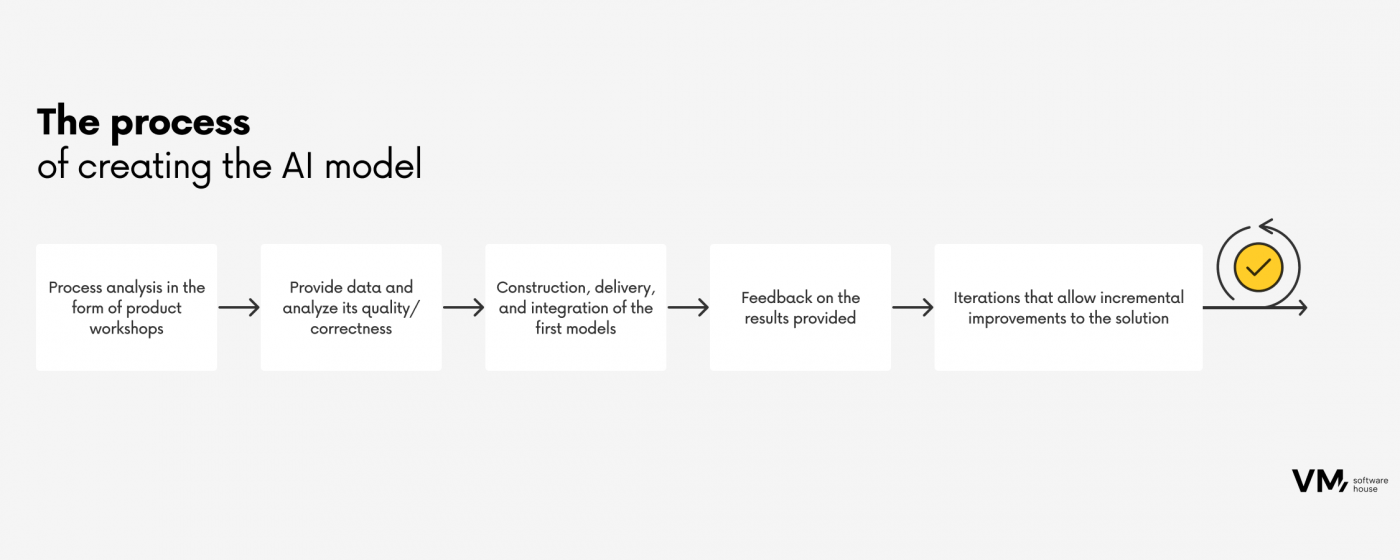
Results
- Customer savings increased by 30% (compared to previous simple models). The improvement in detection rate in the first few months was 60%.
- The percentage of actual fraud in the group of withdrawal requests suspected of being fraudulent exceeded 80%.
- The indicator determining the investment’s (ROI) effectiveness took less than two months.
- Predictive models were responsible for 30-40% of the final financial results.
Technologies
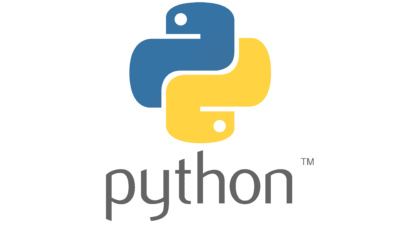
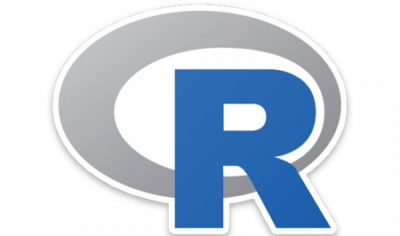
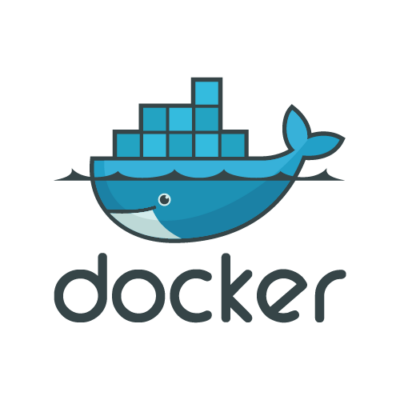
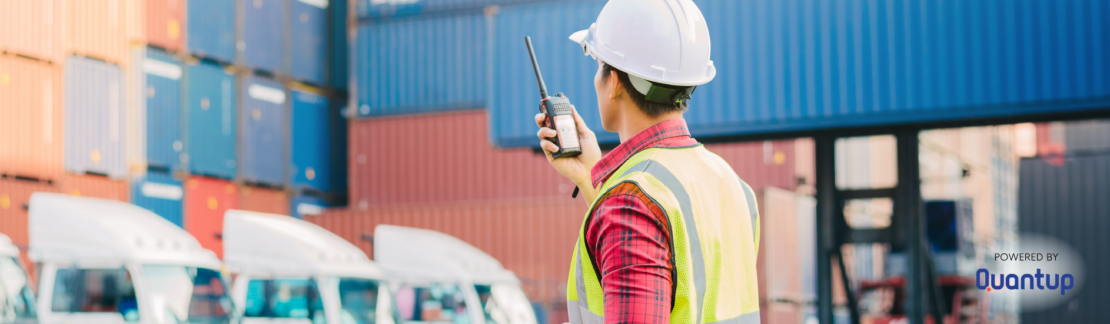
Design, Development, DevOps or Cloud – which team do you need to speed up work on your projects?
Chat with your consultation partners to see if we are a good match.
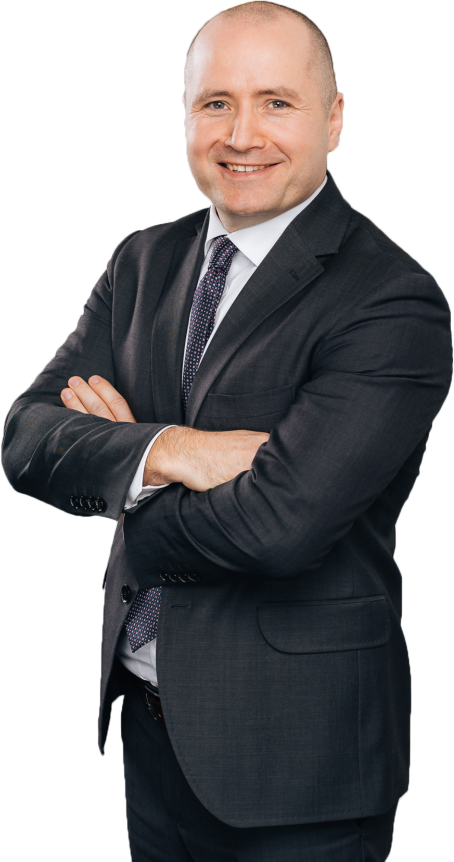