This website uses cookies so that we can provide you with the best user experience possible. Cookie information is stored in your browser and performs functions such as recognising you when you return to our website and helping our team to understand which sections of the website you find most interesting and useful.
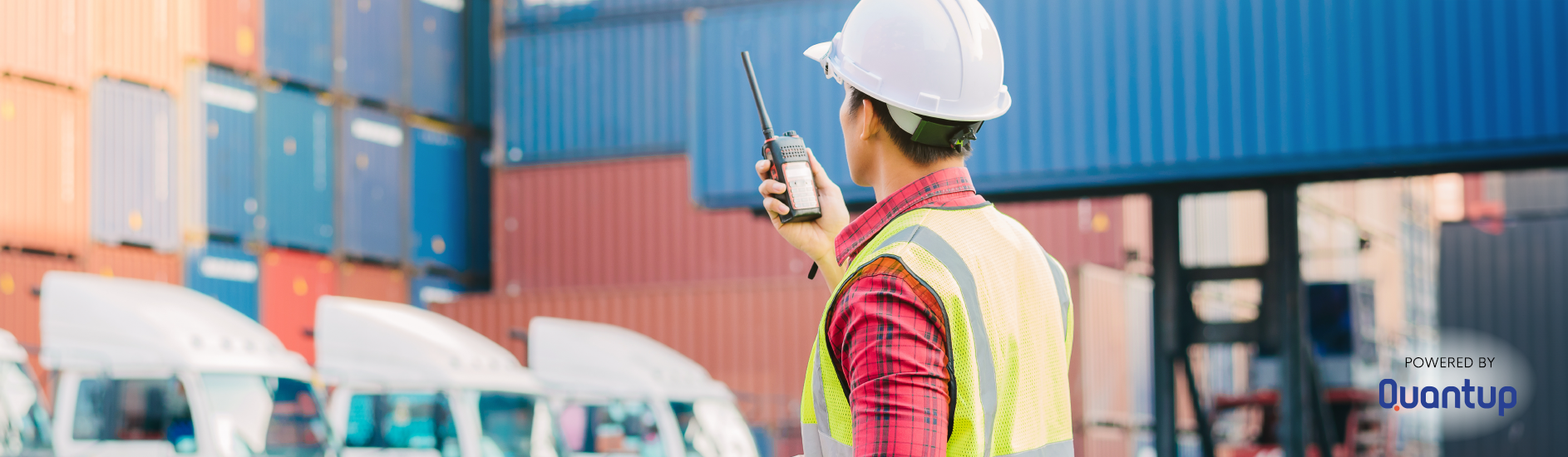
Customer
The client is a company engaged in taking care of the financial security of companies in the transport industry in domestic and international shipping. The company’s specialties include investigating the credibility of contractors, invoice financing and payment monitoring, amicable debt collection, and judicial debt collection.
Challenge
The process of credit risk assessment and decision-making in factoring is a key area of the client’s business. It is important to achieve high performance consistently.
Until now, each application was verified using expert rules and manual inspection. Such an operation was not optimal, as it was difficult to make a completely objective evaluation. Moreover, rapid scaling of the process was impossible. As a result, the client decided to automate the evaluation process for end customers.
Solution
The client decided to cooperate with us in developing software to assess the reliability of end customers. The deciding factor was our extensive experience in fintech projects (for factoring companies, debt collection companies, and banks) and years of experience applying various mathematical models to solve a variety of problems.
The model development process involved five people (senior data analysts, data analysts, programmers) under the supervision of a domain expert.
1. Data analysis and preparation
- A workshop to analyze business needs
- Analysis of the underwriting process, i.e., actions to assess, accept, or reject risks
- Preparation and preliminary analysis of the data collected during this process. At this stage, we focused on understanding the characteristics of the data, the connections between different data sets, and assessing (and improving) the quality of the data.
2. Creation of a suitable ML model and integration
- To implement the solutions, we decided to use Black Box ML models equipped with an explanatory mechanism (XAI).
- We prepared a large set of sample data and carried out the process of learning the model.
- When used, the finished ML model receives the actual data, processes it, and provides the result – a risk assessment.
- Subsequent models were gradually refined. The final models were thoroughly validated, assessing the generalization power of the model and the sensitivity of the prediction quality to the passage of time.
- The models were integrated into the information system at the end of the process.
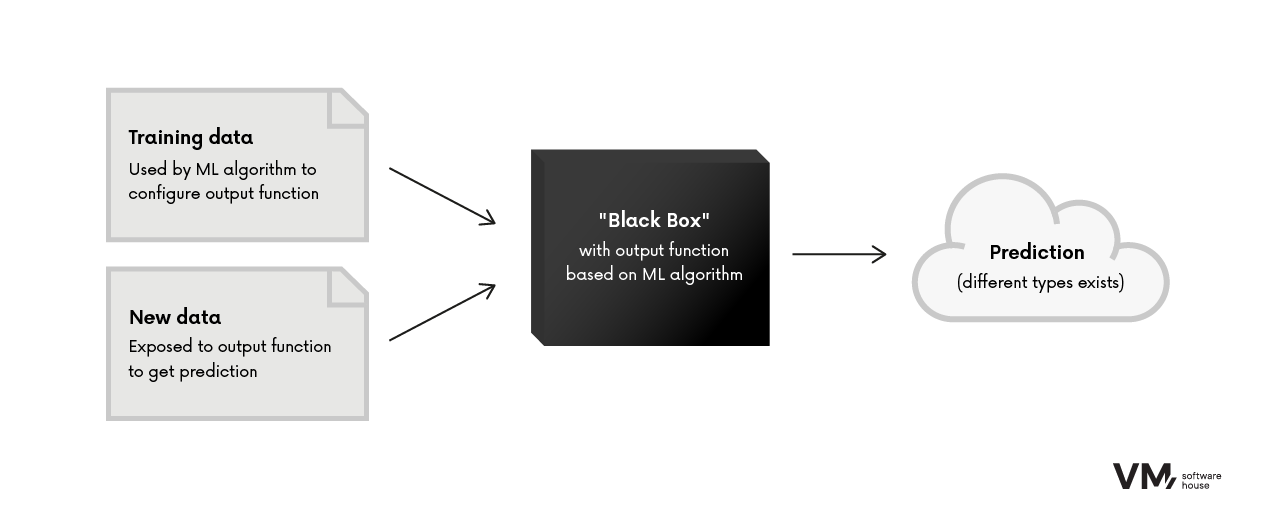
Results
We guided the client through the project, anticipating any emerging risks and proposing alternative methods for solutions. The client was satisfied with the close cooperation, which resulted in high work efficiency. Thanks to open communication, we were able to discuss difficult topics in an accessible way so that all stages of the project and decisions made in the area of predictive modeling could be understood.
In the end, we enabled automatic, objective, and fast evaluations of factoring applications 24/7, which is of great value to end customers. The client also gained the ability to conveniently control risk, independent of the specialists currently reviewing applications, and the ability to scale.
Technologies
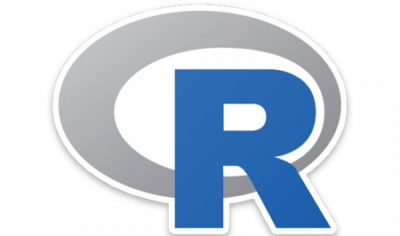
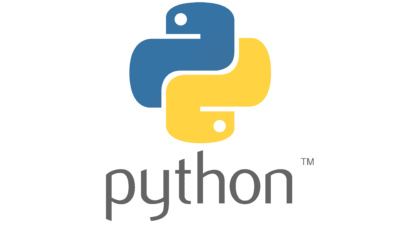
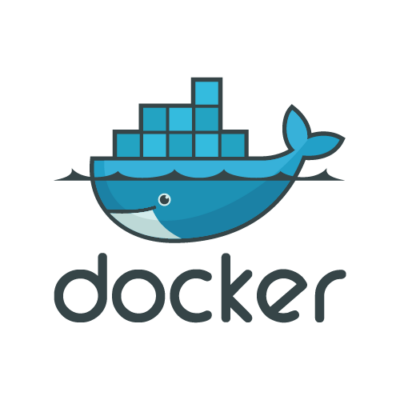
Design, Development, DevOps or Cloud – which team do you need to speed up work on your projects?
Chat with your consultation partners to see if we are a good match.
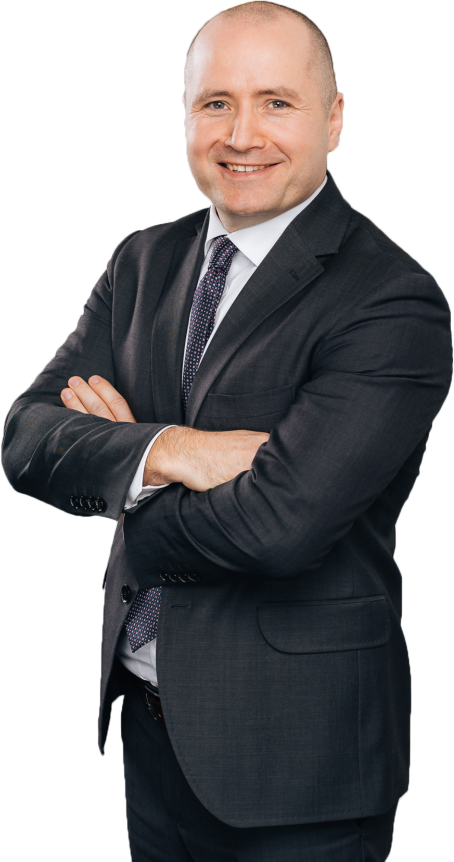