This website uses cookies so that we can provide you with the best user experience possible. Cookie information is stored in your browser and performs functions such as recognising you when you return to our website and helping our team to understand which sections of the website you find most interesting and useful.
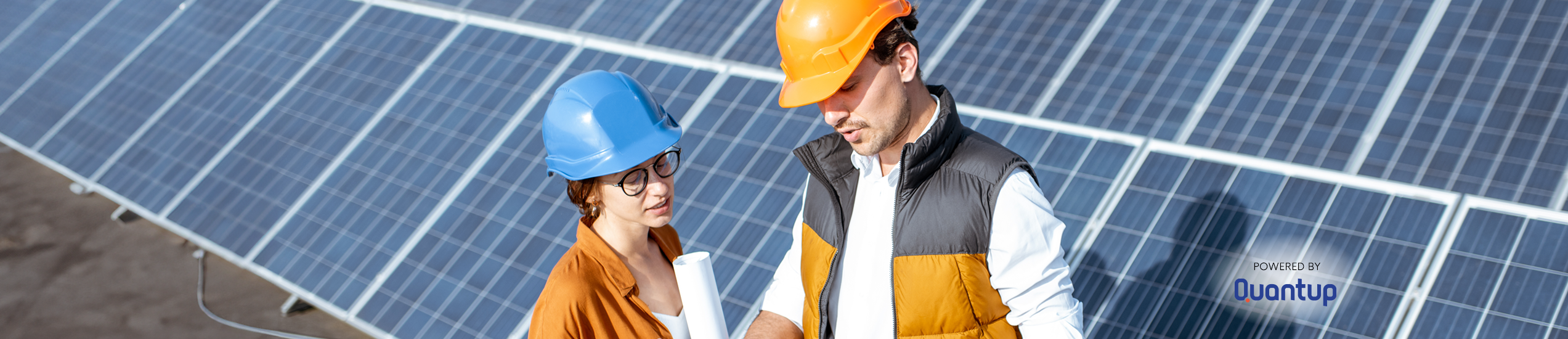
Customer
The client is a UK-based company providing solutions to optimize energy consumption and generation. With market-leading EMS systems, cost-effective solar and BESS systems, and the ability to design and implement custom renewable projects, the client aims for zero CO2 emissions, where every business and community can generate and use its renewable energy.
Challenge
The customer has a system that intelligently decides how electricity is used at the facility. As a result, if the end customer has a photovoltaic or battery installation, it can buy energy from the grid, switch to renewable energy sources, and store or sell the power generated.
The client needed support in creating predictive models that, in the next step, would enable optimization of energy consumption to minimize costs or CO2 emissions, depending on the chosen objective, by making optimal decisions about the energy produced and taken from the power grid: whether to consume, buy, sell or store.
Solution
Our approach consisted of three main stages:
1. Analyze historical data
At this stage, we used data from end-customer installations to design predictive algorithms.
2. Propose a solution for building predictive models.
During the project, we considered classical and ML models in simple and complex variants. We analyzed the dependence of energy consumption and generation on time.
The solution consists of the following components:
- Electricity consumption forecast module
- Electricity production forecast module
- Optimization module that decides whether to use energy from specific sources (grid/photovoltaic), consume, store, buy, or sell, depending on demand and electricity prices
For each prediction, we developed appropriate mathematical methods tailored to the specific situation, depending, among other things, on the data size. As a result, we selected models with an optimal compromise between complexity and accuracy. The optimization module is tailored to customers’ system use cases.
3. Build software and test predictive models.
We designed and built a solution capable of building predictive models tailored to each institutional client separately. Based on the predictions provided by the models, the optimizer proposes the optimal handling strategy. Our data scientists have validated the solution carefully.
Results
We completed the project on time and on budget, and the accuracy of the solution’s performance was in line with the client’s expectations. Thanks to close communication with the client, we quickly understood their business priorities, which facilitated project management. It positively impacted the speed of delivery and achievement of the client’s goals.
Technologies
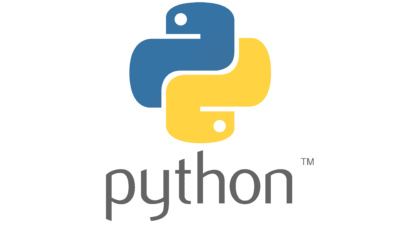
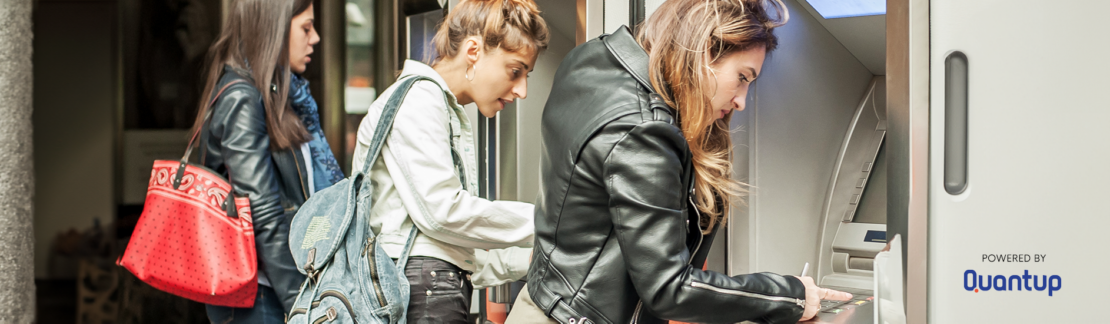
Design, Development, DevOps or Cloud – which team do you need to speed up work on your projects?
Chat with your consultation partners to see if we are a good match.
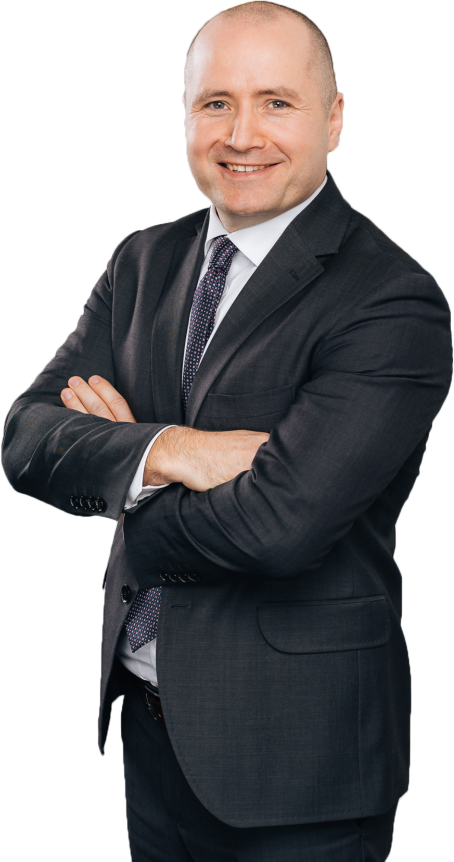