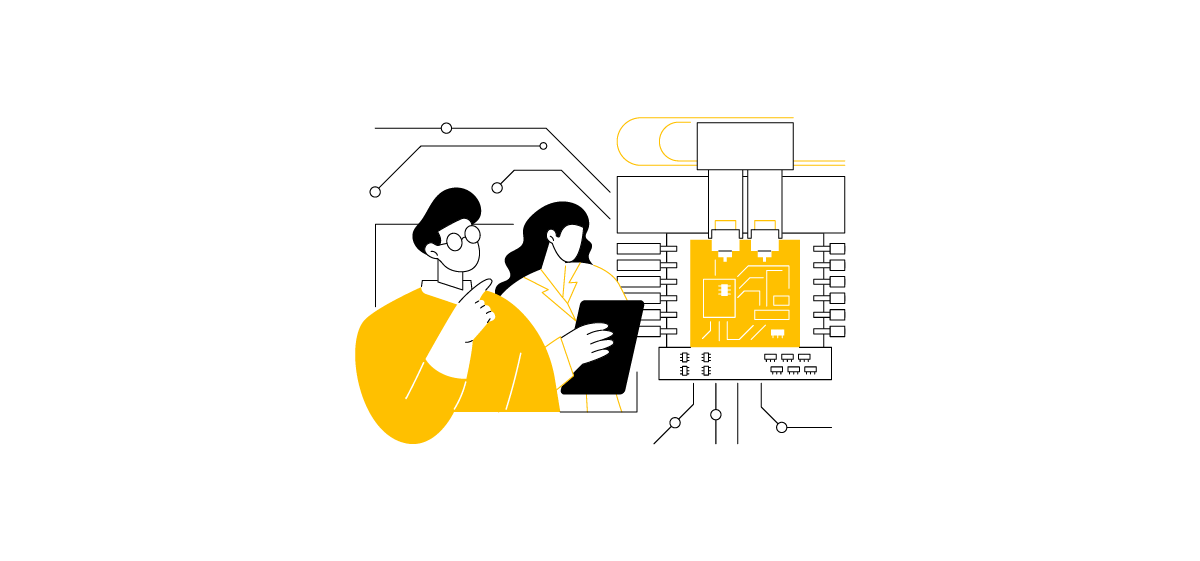
Data is paramount in the modern business landscape. Companies depend on it for decision-making, trend spotting, and strategy development. To maintain a competitive edge, organizations must grasp both the nuances of their internal operations and the fluctuating dynamics of the market. Data analysis and risk assessment complexity is growing, necessitating sophisticated approaches and technologies. Artificial intelligence (AI) is at the forefront, transforming data analysis techniques.
A Gartner Inc. survey from July 2023 reveals that 79% of corporate strategists consider technologies such as analytics, artificial intelligence (AI), and automation vital for their success in the coming years. They estimate that, on average, 50% of strategic planning and execution activities could be partially or fully automated, a significant leap from the current 15%.
AI’s impact spans a broad range of industries, including finance, healthcare, and cybersecurity. Its data analysis tools are adept at identifying outliers, whether in medical diagnoses, financial fraud detection, or product quality assurance. This capability allows organizations to pinpoint potential risks, forecast future performance, and enhance overall operational efficiency. AI’s continuous learning and adaptability are proving indispensable for strategic decision-making, risk mitigation, and securing competitive market advantages.
This article delves into how AI is revolutionizing data analysis and risk assessment, examining its applications, benefits, and the promising future of this disruptive technology.
Table of Contents
Traditional Data Analysis vs. AI
Accurate data and risk analysis are crucial for strategic decision-making and risk mitigation. Insights into market trends, customer behaviors, and needs, and potential business threats are derived from data. Traditional data analysis methods, often bogged down by manual inputs, sorting, and analysis, were time-consuming and prone to human errors. These methods fall short in today’s dynamic business environment.
AI algorithms now enable companies to automate and refine numerous processes. Data analytics involves examining raw data (including structured and unstructured data) and revealing patterns, relationships, and trends critical for informed decision-making. Risk analysis, meanwhile, identifies and assesses potential threats to business performance or objectives. By merging these disciplines, companies can comprehensively understand their operations, identify risks, and devise strategies for their mitigation.
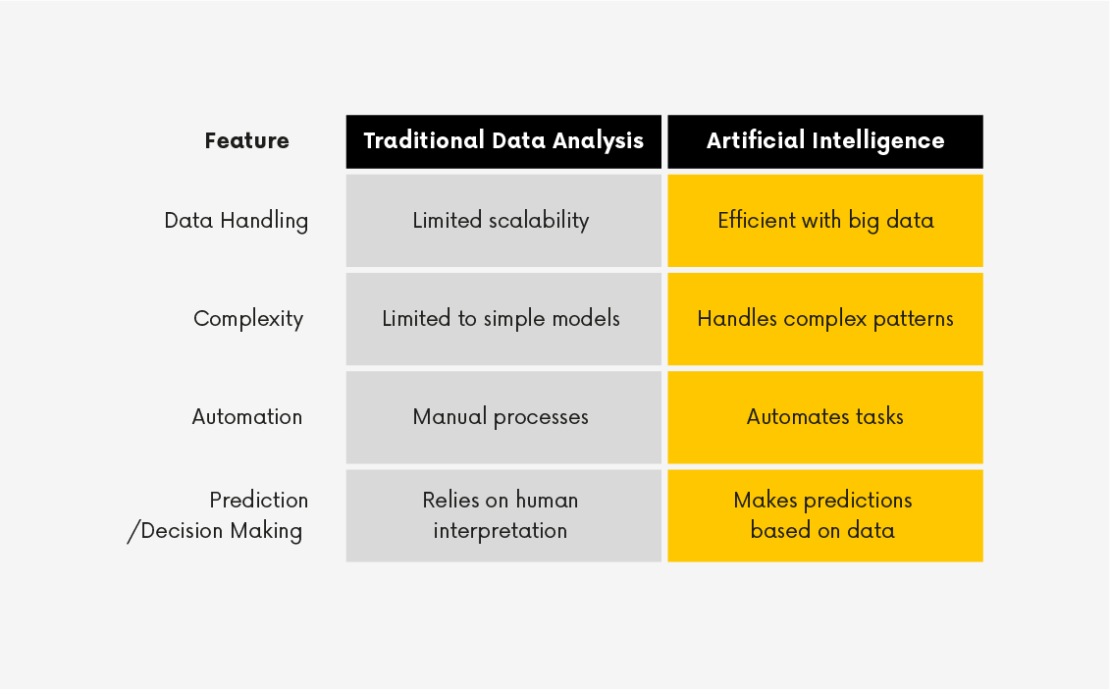
Data Analysis Process Enhanced by AI
The implementation of AI frequently involves the creation of a mathematical model, integral to the software utilized in the processes discussed previously. We will explore the iterative phases of the data analysis process that lead to the development of such models. These steps are foundational for working with data across various domains, including business intelligence, data visualization, research, and machine learning.
1. Defining the Target
Initially, determine the business issue you aim to address. Formulate a hypothesis and devise a method for testing it.
2. Definition of KPIs
Decide on the criteria for evaluating the success of your approach. This could involve, for example, the algorithm’s error rate and the minimum performance threshold required to meet your objective.
3. Collecting Data Related to the Problem
Gather existing data from various sources such as databases, APIs, spreadsheets, or other sources. Ensure the data types are comprehensive and accurately reflect the problem at hand. Verify your access to these data sources and any consent needed for their use.
4. Data Cleaning
Different types of data often arrives unstructured, incomplete, or inconsistent and must be preprocessed. This involves handling missing values, eliminating duplicates, and addressing outliers. The complexity of data acquisition and preparation typically consumes up to 80% of an AI project’s timeline.
5. Exploratory Data Analysis (EDA)
Exploratory Data Analysis (EDA) is a critical stage where we delve into the data to understand its distributions, correlations, and patterns. EDA aims to grasp the data’s structure and unearth potential relationships. The process initiates with the generation of automated reports detailing the data’s various characteristics. However, complete automation is challenging. This step requires human verification of the data’s significance and accuracy, often involving consultations with individuals well-acquainted with both the data and the related business processes. Neglecting or oversimplifying data preparation at this stage could lead to costly errors.
6. Pre-Selection of Features
The goal here is to identify data features (variables) with a significant impact on the problem at hand and to eliminate those that are irrelevant. Feature selection not only simplifies the model but also enhances its performance. It’s important to note that most business challenges do not necessitate complex models. This phase focuses on selecting potentially useful features and excluding definitively non-useful ones, leaving the final selection to machine learning (ML) algorithms.
7. Enabling ML Algorithms
This step involves choosing suitable machine learning algorithms based on the problem type (classification, regression, clustering, etc.), as well as considering the data’s type, complexity, size, and the model’s speed requirements. The model is then trained and fine-tuned using prepared data. Typical algorithms include linear and nonlinear regression, decision trees, boosted trees, neural networks, among others.
8. Model and Hypothesis Testing
Data points are divided into training and test sets to test the model and hypotheses. The model’s performance is assessed using various metrics, and adjustments are made to the hyperparameters (i.e., parameters that control model complexity) to fine-tune the model.
9. Implementation of the Model
For predictive models, implementation in a real-world setting is essential. This involves integrating the model into applications, websites, or business processes. It’s crucial to acknowledge that a model’s operation within a business context may differ from its performance in a testing environment, which is a natural occurrence.
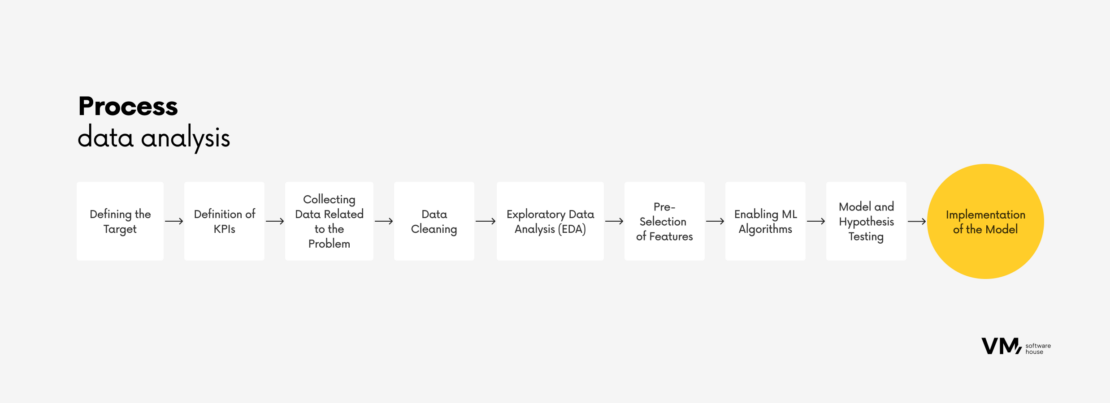
Although described as sequential steps for simplicity, the process is more iterative in reality. For instance, discovering incorrect data necessitates returning to the preparation and analysis phases.
The discussion has primarily focused on the standard procedure for developing supervised models, such as evaluating the likelihood of default using historical repayment data.
Next, we will explore three scenarios where artificial intelligence offers profound insights into data and business risk analysis.
1. Data Collection and Analysis
Artificial intelligence, through algorithms, machine learning (ML), and natural language processing (NLP) techniques, excels in swiftly identifying trends, patterns, and anomalies that might remain undetected by traditional methods.
Real-Time Data Analysis
AI’s capability to process vast volumes of data far surpasses human capacity, enabling real-time data analysis and timely decision-making. This rapid processing allows companies to foresee potential threats and implement preventative strategies effectively.
Using Big Data to Collect and Analyze Data
ML algorithms empower organizations to handle and scrutinize large datasets in real-time, effortlessly navigating through extensive information to reveal patterns and relationships invisible to the human eye. AI’s analysis of big data sets uncovers hidden connections and trends, delivering crucial insights for informed decision-making.
Predictive Analysis and Forecasting
AI algorithms stand out in forecasting future performance with remarkable precision. By analyzing past data and employing ML techniques, AI can accurately forecast upcoming trends, customer behaviors, and potential risks.
NLP – Natural Language Processing
AI’s ability to process various unstructured data types, such as text, images, and videos, which are often challenging for humans to analyze, marks a significant advancement. NLP enables AI to derive meaningful insights from textual data, facilitating sentiment analysis, theme discovery, and other sophisticated analyses.
Amazon is one example of companies investing in AI for research and development. It leverages AI for diverse functions, including personalized recommendations, optimizing supply chains, and enhancing its Alexa devices through NLP.
2. Personalizing Financial Services
Data science and artificial intelligence are pivotal in the personalization of financial services. Below, we explore their synergy in crafting bespoke customer experiences:
Personalized Recommendations of Products and Services
Through the analysis of extensive customer data, including behavioral patterns and transaction histories, AI tailors product suggestions to match individual preferences and feedback. This personalized approach enhances customer satisfaction and engagement.
AI Chatbots for Better Customer Service
AI chatbots are revolutionizing customer service. Whether it’s checking an account balance, understanding the terms of a mortgage, or troubleshooting a problem, AI chatbots are streamlining customer interactions. Chatbots handle routine inquiries, navigate users through complex processes, and provide customized advice. For more complex issues, chatbots efficiently direct customers to human consultants, ensuring a seamless service experience.
Create Customer Profiles for Risk Assessment
By constructing detailed customer profiles from historical data, AI identifies patterns indicative of high-risk behaviors. This enables financial institutions to refine their risk management strategies, such as adjusting credit limits or flagging unusual transactions, using either predictive models (supervised methods) or micro-segmentation (unsupervised methods) for nuanced risk assessment.
Real-Time Monitoring and Insight
Artificial intelligence offers continuous monitoring of accounts, transactions, and user behavior, swiftly identifying anomalies or potential fraud. These real-time insights allow financial institutions to promptly protect their customers and assets. Additionally, AI can detect early signs of customer churn, providing an opportunity to retain the customer.
Customized Marketing Campaigns
AI plays a crucial role in devising targeted marketing campaigns. Whether offering personalized credit rates or suggesting appropriate insurance policies, AI ensures that marketing messages to these segments are significantly enhanced by AI. In each of these areas, the integration of data science and artificial intelligence not only streamlines operations but also significantly improves the customer experience in the financial services sector.
3. Using Data to Detect Fraud
Financial fraud has evolved into a sprawling, multi-billion-dollar menace in our increasingly digital world. The Nilson report forecasts that credit card losses globally will surge to $43 billion by 2026. To counteract this threat, financial services firms are progressively adopting AI-based solutions for fraud detection. These advanced tools empower institutions to outpace cybercriminals, safeguard assets, and preserve customer trust.
Here’s how artificial intelligence is being used to detect fraud in real-time:
Machine Learning Models
AI-driven fraud detection leverages a suite of machine learning models to scrutinize data integrity, customer behavior, relationships, and account patterns for anomalies. These models are adept at pinpointing traits indicative of fraudulent activities. Key capabilities include:
- Real-Time Reaction: Continual transaction monitoring to identify potential red flags.
- Enhanced Detection Accuracy: Utilizing deep learning and neural networks to refine fraud detection precision.
- Behavior Analysis: Employing behavioral biometrics to study user interactions.
- Entity Relationship Investigation: Applying graph analytics to explore connections between various entities (such as customers, accounts, transactions) in the hunt for coordinated fraud schemes.
Generative Artificial Intelligence
Generative AI and large language models (LLMs) herald unprecedented opportunities across numerous sectors, including financial services. These technologies can, for instance, be harnessed to create more intelligent and efficient chatbots, significantly enhancing customer interaction and service delivery in the financial domain.
Case Study: How Machine Learning Algorithms Improved Detection of Suspicious Claims by 80%
In the insurance industry, distinguishing between legitimate claims and fraudulent attempts is paramount. For a client within this sector, we developed a sophisticated model designed to significantly outperform its predecessors in identifying the veracity of claims. This advancement leveraged algorithms rooted in historical data, complemented by comprehensive process and data analysis methodologies.
The results were strikingly quantifiable. Post-implementation, the client recorded a 30% increase in savings compared to the outcomes with earlier, simpler models. More impressively, the new model enhanced the detection of suspicious claims by over 80%. This case exemplifies the profound impact that machine learning algorithms can have on fraud detection processes, offering substantial improvements in efficiency and financial performance.
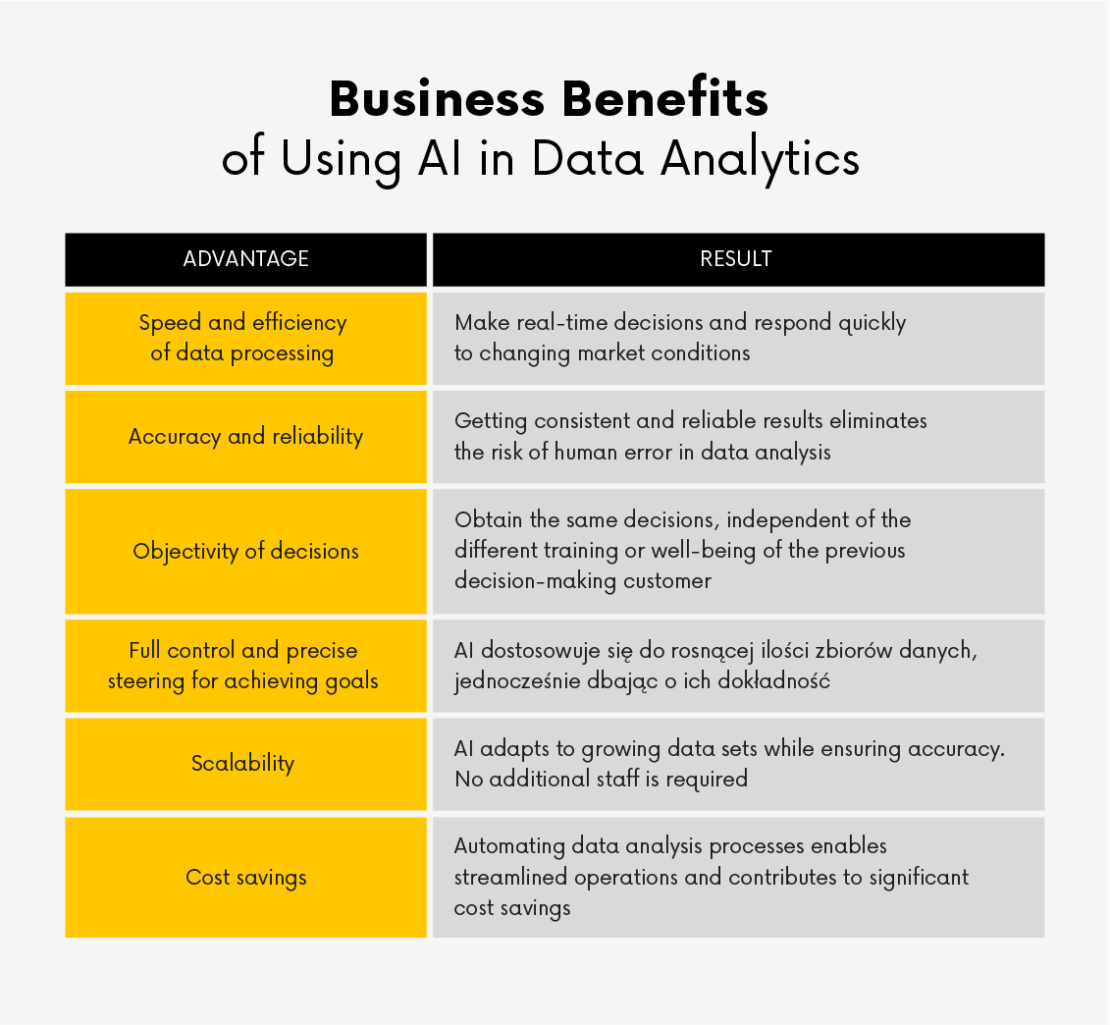
Future Trends and Opportunities in Artificial Intelligence-Based Data and Risk Analysis
Investment in AI research and implementation is on an upward trajectory. A Statista analysis revealed that global spending on artificial intelligence (AI), encompassing software, hardware, and services for AI-oriented systems, surged to $154 billion in 2023, marking a 26.9% increase from 2022. The spotlight was on artificial intelligence and data science, significantly due to the advent of Generative AI, highlighted by innovations such as OpenAI’s ChatGPT, Google’s Bard, and the recent introduction of Gemini, a multimodal AI system.
As AI development progresses, certain trends are poised to influence corporate evolution:
- Shifting Investments in Generative AI: As generative AI advances, businesses must adjust their processes, enhance workforce skills, and ensure seamless integration with existing infrastructure.
- Ethical and Responsible AI Practices: There’s a growing recognition of AI’s ethical implications. Expect a heightened focus on establishing ethical guidelines, detecting biases, and fostering responsible AI practices.
- AI’s Crucial Role in Risk Management: Predictive analytics, anomaly detection, and natural language processing (NLP) will become essential in spotting and mitigating risks across various sectors, including finance, healthcare, and cybersecurity.
- Synergy of Edge AI and IoT: Implementing AI models in edge computing (directly on devices or sensors) facilitates real-time processing and minimizes latency. This synergy, combined with the Internet of Things (IoT), will pioneer new avenues for data insights.
Transform Your Business With AI Data Analytics
Artificial intelligence is redefining the approach to data analysis and risk mitigation. Leveraging AI algorithms enables businesses to sift through vast datasets in real time, pinpoint potential risks, and accelerate decision-making processes.
At VM.PL, we are enthusiastic about the fusion of artificial intelligence and analytics. It’s a common misconception that AI operates autonomously; like any technological solution, it requires customization to meet specific needs. Expert support is crucial. Our team of data scientists is equipped to empower your business with AI, propelling you to new developmental heights. Interested in exploring the capabilities of your own AI analytics platform? Let’s talk about how we can address your business needs.