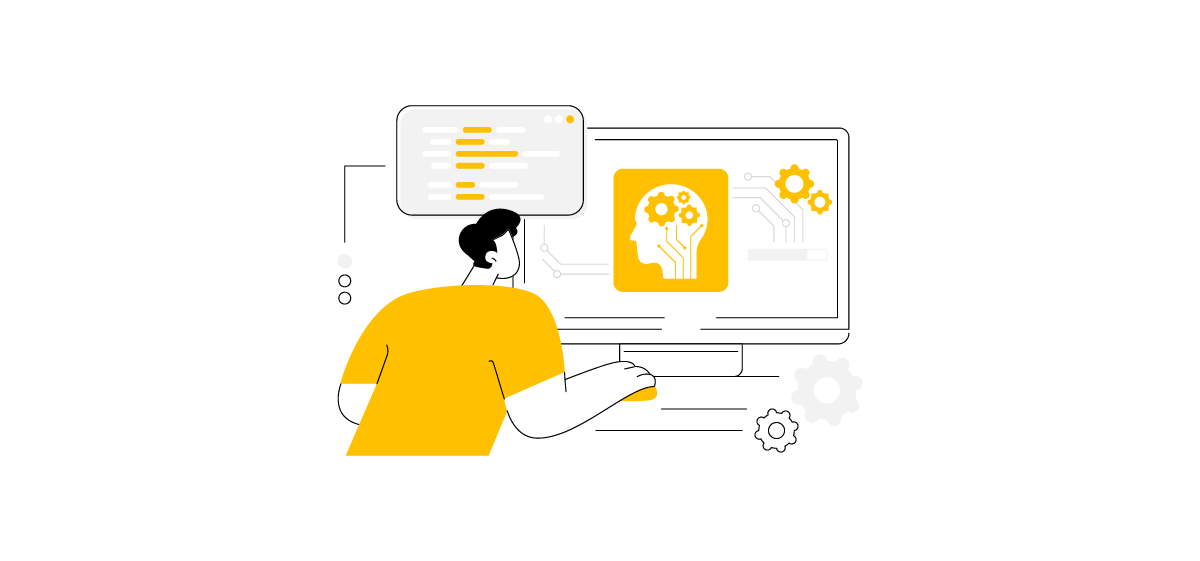
/ 18.11.2024
Real-time data insights, faster time-to-market, and continuous monitoring—the benefits of using digital twins for simulation are numerous, provided the right conditions are in place. But which technology is better suited for your project: simulations or digital twins?
Though the idea of simulators and digital twins may seem cutting-edge, the concept has been around for some time. For instance, during the Apollo 13 mission in the 1960s, ground engineers were able to safely bring the astronauts back to Earth after an oxygen tank explosion. They did this by simulating a carbon dioxide filter replacement, which the astronauts then recreated on board.
As technology advances, businesses now leverage simulations and digital twins to optimize processes, test new ideas, and predict future outcomes. By developing digital twins, companies can improve product development, streamline operations, and enhance customer engagement through enriched experiences and interactions.
In fact, in 2019, digital twins were identified as one of the top ten strategic technologies that significantly improved decision-making processes for businesses. A McKinsey study reported that 70% of technology executives at large companies are investing more than $48 billion in digital twin technologies, with an anticipated compound annual growth rate of 58% by 2026.
This article delves into the core differences between simulations and digital twins to help you decide which solution is best for managing your projects.
Table of Contents
A simulation is a virtual model of a process, system, or environment that allows you to test various scenarios without the risks and costs of using actual machinery. Simulations are primarily used for “what-if” analysis, creating a controlled environment where variables can be manipulated to observe outcomes.
Key characteristics of simulations include:
For decades, simulations have been widely used across industries to analyze how different factors impact processes. Automotive engineers, for example, use crash simulations to understand how vehicles behave during collisions. Medical professionals utilize patient simulators for performing complex surgeries, and economists create financial simulations to forecast the economic effects of certain policy decisions.
A digital twin is more than just a model—it’s a dynamic, virtual representation of a physical object, system, or process that is continuously updated with real-world data. Digital twins enable companies to optimize performance, predict future issues, and make real-time adjustments to operations.
Digital twins are powered by advanced technologies such as Internet of Things (IoT) sensors, artificial intelligence (AI), machine learning (ML), cloud computing, and data analytics. They are constantly connected to real-time data sources, often through embedded sensors in their physical counterparts, which feed live data back to the model to ensure synchronization with reality.
Key features of digital twins include:
In the manufacturing industry, for instance, digital twins are used to monitor equipment and predict maintenance needs before a machine fails. Similarly, digital twins of human organs are being used in healthcare to simulate personalized treatments.
Simulations are ideal when you need to test multiple hypothetical scenarios or conditions over time but don’t require real-time data. This is particularly useful for new product designs in a controlled virtual environment without building physical machines.
Simulations allow you to model various conditions, such as extreme heat or pressure, to evaluate product performance. They also enable you to test multiple scenarios to identify optimal solutions during product development.
When should simulations be used to test scenarios in complex systems?
For a client specializing in machinery manufacturing, we developed a project to simulate the production environment, aiming to reduce costs associated with implementing a new product for the end customer. This technological process allows us to detect errors early and better understand the impact of changes on the production process.
The creation of a basic simulation module involved five steps:
Simulations typically rely on static data sets or predefined inputs, meaning they lack live updates. However, a digital twin is the better solution if your project requires continuous feedback from the physical system—such as in manufacturing or smart cities where real-time monitoring is crucial.
A true digital twin works throughout the product life cycle (SDLC), from design to service, offering a more integrated solution across the entire process chain. The key benefits of implementing digital twins include:
However, implementing digital twins requires digital maturity within the organization. This includes a robust data infrastructure, such as PLMs (Product Lifecycle Management), PDMs (Product Data Management), and access to high-quality data from testing and real-world production environments.
When speaking with managers from various companies, three key challenges often emerge when implementing a digital twin:
1. Cost
Significant resource investment is required from the outset to achieve value. Building a digital twin demands larger investments, including IoT devices, cloud infrastructure, and data management tools.
2. Skills:
Implementing a digital twin requires:
3. Data Quality: Digital twins depend on vast amounts of real-time data from sensors, which takes time to gather and requires highly skilled resources to manage and integrate.
Given these challenges, companies need to determine the most appropriate use case for starting digital twin development. The following steps can help ensure a successful project:
Choosing between a simulation and a digital twin depends largely on your project’s specific goals and needs. If you require one-time analysis, scenario testing, or training, a simulation is likely the best option. However, a digital twin would be a more effective solution if your project demands real-time monitoring, continuous optimization, and data-driven decision-making.
Understanding these key differences will help you select the right tool, maximizing the technology’s impact on your project’s success. If you’d like to discuss your project needs, our experienced specialists can answer your questions and provide guidance on the best solutions.
/ Latest information